Learning social skills next target for AI
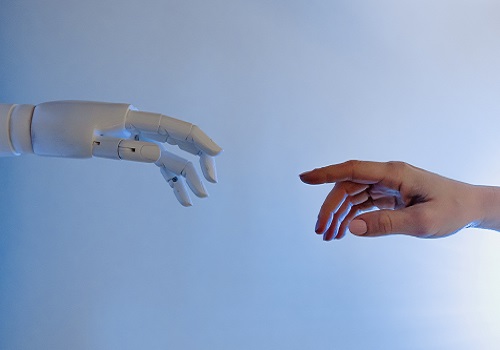
Follow us Now on Telegram ! Get daily 10 - 12 important updates on Business, Finance and Investment. Join our Telegram Channel
https://t.me/InvestmentGuruIndiacom
Download Telegram App before Joining the Channel
Siri and Google Assistant may be able to schedule meetings on request, but so far they don't have the social understanding to independently prioritise the appointments.
According to researchers based in China, Artificial Intelligence (AI) is smart, but it is stunted by a lack of social skills.
"Artificial intelligence has changed our society and our daily life," first author Lifeng Fan, from Beijing Institute for General Artificial Intelligence (BIGAI) said.
"What is the next important challenge for AI in the future? We argue that Artificial Social Intelligence (ASI) is the next big frontier," Fan said.
In a paper, published in the CAAI Artificial Intelligence Research, the team explained that ASI comprises multiple siloed subfields, including social perception, theory of Mind -- the understanding that others think from their own point of view -- and social interaction.
By using cognitive science and computational modelling to identify the gap between AI systems and human social intelligence, as well as current issues and future directions, Fan said the field will be better equipped to advance.
"ASI is distinct and challenging compared to our physical understanding of the work; it is highly context-dependent," Fan said.
"Here, context could be as large as culture and common sense or as little as two friends' shared experience. This unique challenge prohibits standard algorithms from tackling ASI problems in real-world environments, which are frequently complex, ambiguous, dynamic, stochastic, partially observable and multi-agent."
Fan said that ASI requires the ability to interpret latent social cues, such as eye-rolling or yawning, to understand other agents' mental states, such as belief and intent, and to cooperate in a shared task.
According to Fan, the best approach is a more holistic one, mimicking how humans interface with one another and the world around them. This requires an open-ended and interactive environment, as well as consideration for how to introduce better human-like biases into ASI models.
"To accelerate the future progress of ASI, we recommend taking a more holistic approach just as humans do, to utilise different learning methods such as lifelong learning, multi-task learning, one-/few-shot learning, meta-learning, etc.," Fan said.
"We need to define new problems, create new environments and datasets, set up new evaluation protocols, and build new computational models. The ultimate goal is to equip AI with high-level ASI and lift human well-being with the help of Artificial Social Intelligence."
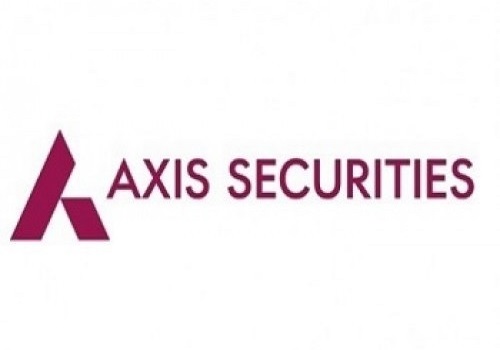
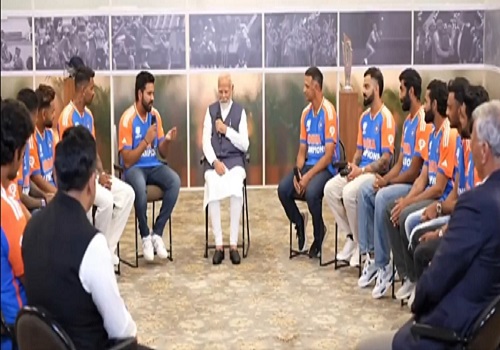
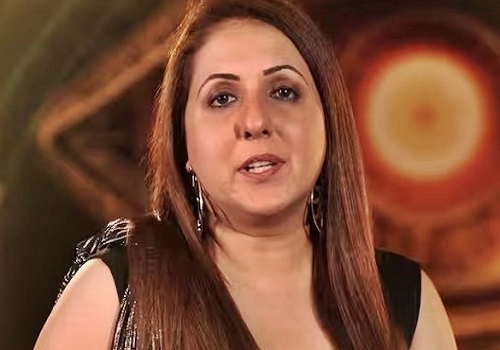
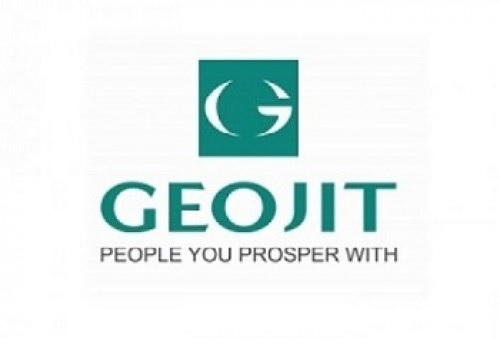
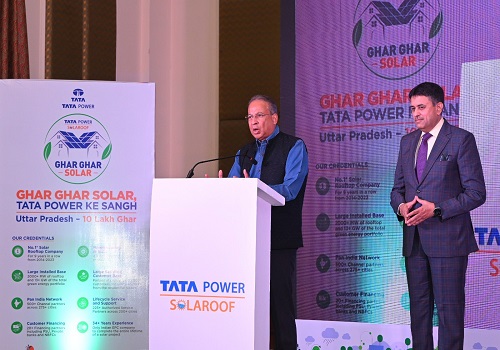
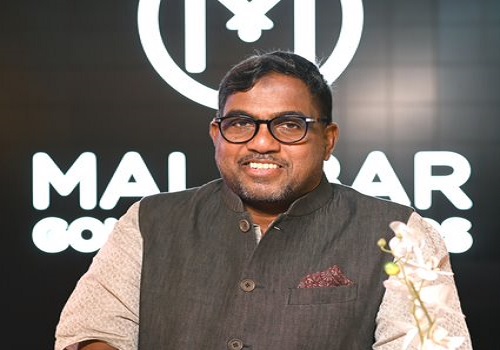
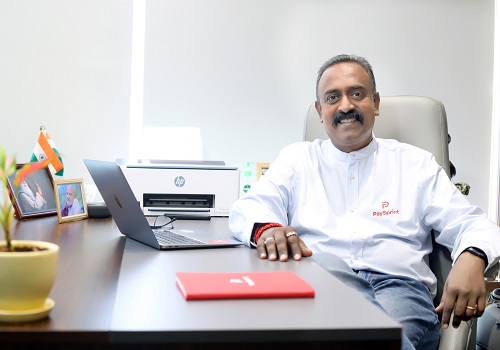
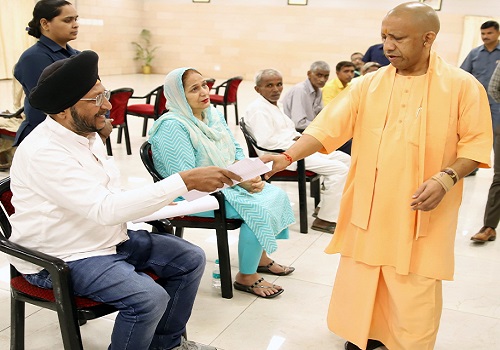
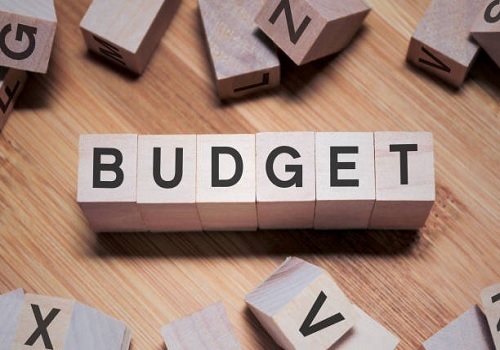
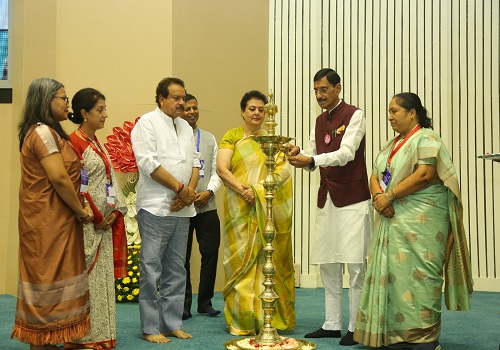